A groundbreaking study conducted by researchers from the Massachusetts Institute of Technology and Brown University has unveiled a promising new application of artificial intelligence (AI) in the prediction of rare natural disasters, potentially outperforming traditional forecasting methods.
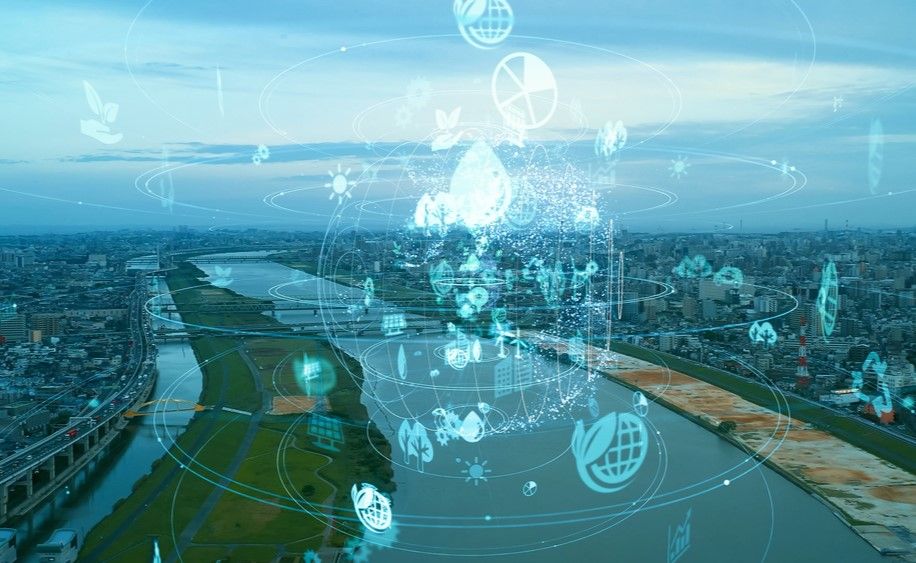
AI-Based Forecasting with Minimal Data
The novelty of this approach lies in the use of advanced machine learning and statistical algorithms that require significantly less data to make accurate predictions about natural disasters such as earthquakes, wildfires, and hurricanes. Given the infrequency of such events, the scarcity of historical data has traditionally posed a challenge to precise forecasting.
George Karniadakis, a professor of applied mathematics and engineering at Brown University, highlights the crux of their research: leveraging the optimal data to reduce the necessity for extensive data points. Through their innovative method, the team aims to utilize existing samples effectively to anticipate future occurrences.
Serial Sampling and Active Learning
The breakthrough came with the implementation of serial sampling coupled with active learning techniques. This approach allows the algorithms to analyze incoming data iteratively, gaining insights and identifying further significant data sets. This method exemplifies the principle that more can be achieved with less, optimizing the use of available information.
DeepOnet: A Neural Network Solution
Central to their method is DeepOnet, an artificial neural network designed to mimic the human brain's neuronal pathways through interconnected nodes. This technology merges the capabilities of two neural networks, enabling the rapid processing and generation of vast amounts of data.
Predicting the Unpredictable
By integrating DeepOnet with active learning strategies, the researchers successfully demonstrated the system's ability to detect precursors to catastrophic events with minimal data. This AI-based approach represents a significant leap forward in the field of natural disaster prediction, offering a more efficient and potentially more accurate alternative to existing forecasting models.
This study not only showcases the potential of AI in enhancing our predictive capabilities for rare natural disasters but also opens new avenues for utilizing machine learning in various scientific disciplines where data scarcity is a challenge.